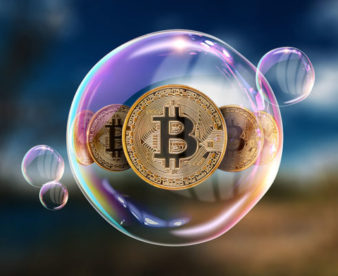
Alt Data / Analytics /
Forecasting
Decisions are increasingly data-driven. ZettaCap helps collect, structure, and analyze many forms of alternative data such as social media, search, job postings, implied foot traffic, SEC filings and others.
ZettaCap leverages alternative data and analytics to forecast product demand, hiring trends, election outcomes, revenue growth prospects, macro trends and others.
Sometimes data manipulation is not as straight-forward as you’d like. ZettaCap creates bespoke cloud-based data gathering, ETL, storage and analysis systems to take the bite out of your project.
Traditional fundamental analysis merges with modern data-driven quantitative analysis to create ‘quantamentals’. ZettaCap leverages its own as well as third party data to help provide deeper insights.
Zettacap uses its proprietary Social Media Influence (SMI) model to forecast elections. ZettaCap published the only correct forecast of the Trump victory and the most accurate forecast of Macron’s win — both out-of-consensus calls.
Improved analysis using new data / forms of analysis creates opportunities to increase alpha for investors; sentiment for trading, job postings for fundamentals, implied foot traffic for revenue, influence rankings, etc.
Multiple press outlets called the state for Biden. However, many have complained…
Read MoreLastly, we will take a look at the House and Senate. In…
Read More